Image Classification: A Survey

DOI:
https://doi.org/10.54060/JIEEE/001.02.002Keywords:
Machine Learning, Deep Learning, Deep Neural Networks, Convolutional Neural Network, CIFAR-10 DatasetAbstract
The Classification of images is a paramount topic in artificial vision systems which have drawn a notable amount of interest over the past years. This field aims to classify an image, which is an input, based on its visual content. Currently, most people relied on hand-crafted features to describe an image in a particular way. Then, using classifiers that are learnable, such as random forest, and decision tree was applied to the extract features to come to a final decision. The problem arises when large numbers of photos are concerned. It becomes a too difficult problem to find features from them. This is one of the reasons that the deep neural network model has been introduced. Owing to the existence of Deep learning, it can become feasible to represent the hierarchical nature of features using a various number of layers and corresponding weight with them. The existing image classification methods have been gradually applied in real-world prob-lems, but then there are various problems in its application processes, such as unsatis-factory effect and extremely low classification accuracy or then and weak adaptive abil-ity. Models using deep learning concepts have robust learning ability, which combines the feature extraction and the process of classification into a whole which then com-pletes an image classification task, which can improve the image classification accuracy effectively. Convolutional Neural Networks are a powerful deep neural network tech-nique. These networks preserve the spatial structure of a problem and were built for object recognition tasks such as classifying an image into respective classes. Neural networks are much known because people are getting a state-of-the-art outcome on complex computer vision and natural language processing tasks. Convolutional neural networks have been extensively used.
Downloads
References
M. Swamynathan,” in Mastering Machine Learning with Python in Six Steps,”Berkeley, CA: Apress, pp. 297–344, 2017.
S. M. D. Lainez and D. B. Gonzales, “Automated fingerlings counting using convolutional neural network,” in IEEE 4th International Conference on Computer and Communication Systems (ICCCS), pp.67-72, Feb 2019.
K. Narmada, G. Prabakaran, S. Mohan,”Classification and stage prediction of lung cancer using convolutional neural networks,” vol. 8, no. 10, pp. 993–998, Aug 2019.
J. Zhang, F. Hu, L. Li, et al, “An adaptive mechanism to achieve learning rate dynamically,” Neural Comput. Appl., vol. 31, no. 10, pp. 6685–6698, 2019.
H. A. Shiddieqy, F. I. Hariadi, and T. Adiono, “Implementation of deep-learning based image classification on single board computer,” in International Symposium on Electronics and Smart Devices (ISESD), pp. 133-137, oct 2017.
S. Singh and N. Singh, “Object classification to analyze medical imaging data using deep learning,” in International Conference on Innovations in Information, Embedded and Communication Systems (ICIIECS), 201 pp. 1-4, March 2017.
K. He, X. Zhang, S. Ren, et al, “Deep residual learning for image recognition,” in 2016 IEEE Conference on Computer Vision and Pattern Recognition (CVPR), pp. 770-778, June 2016.
N Srivastava, G Hinton, A Krizhevsky, et al,”Dropout: a simple way to prevent neural networks from overfitting.” The journal of machine learning research, vol.15, no.56, pp.1929-1958, 2014.
S. Srinivas, R. K. Sarvadevabhatla, K. R. Mopuri, N. Prabhu, S. S. S. Kruthiventi, and R. V. Babu, “A taxonomy of deep convolutional neural nets for computer vision,” Front. Robot. AI, vol. 2, no.36, pp.1-15, Jan 2016.
A. Krizhevsky, G. Hinton,” Learning multiple layers of features from tiny images.” Technical Report TR-2009, University of Toronto, Toronto, April 2009.
Y.Yang & T.M. Hospedales, “Deep neural networks for sketch recognition”, 2015.
D. Ciregan, U. Meier and J. Schmidhuber, “Multi-column deep neural networks for image classification,” in IEEE Conference on Computer Vision and Pattern Recognition, pp.3642-3649, June 2012.
D. C. Cires, U. Meier, J. Masci, et al,” Flexible, High Performance Convolutional Neural Networks for Image Classification,” IJCAI, pp. 1237-1242 ,2011.
K. Chauhan, S. Ram “Image Classification with Deep Learning and Comparison between Different Convolutional Neural Network Structures using Tensorflow and Keras,” International Journal of Advance Engineering and Research Development, vol. 5, no.2, pp. 533-538, 2018.
S. Khan and S.-P. Yong, “A deep learning architecture for classifying medical images of anatomy object,” in 2017 Asia-Pacific Signal and Information Processing Association Annual Summit and Conference (APSIPA ASC), pp. 1661-1668, Dec 2017.
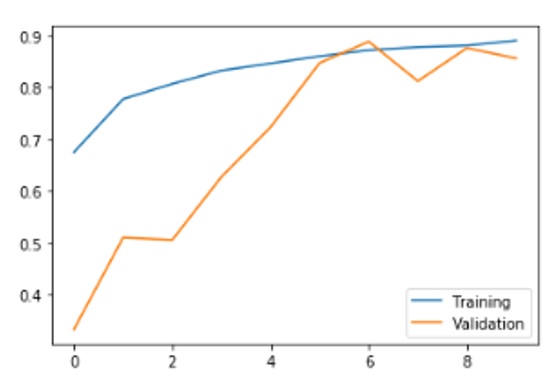