Interpretation and localization of Thorax diseases using DCNN in Chest X-Ray

DOI:
https://doi.org/10.54060/JIEEE/001.01.001Keywords:
Image Processing, Chest Radiography, DCNN, Machine LearningAbstract
In recent years, the use of diagnosing images has been increased dramatically. An entry level task of diagnosing and reading Chest X-ray for radiologist but they ought to re-quire a good knowledge and careful observation of anatomical principles, pathology and physiology for this complex reasonings. In many modern hospital’s the tremendous number of x-ray images are stored in PACS (Picture Archiving and Communication Sys-tem). The conditions of plethora been diagnosed by the sustainable number of chest X-Ray. Our aim to predict the thorax disease categories through deep learning using chest x-rays and their first-pass specialist accuracy. In a paper the main application that present a pathology localization framework and multi-label unified weakly supervised image classification that can perceive the occurrence of afterward generation of bound-ing box around the consistent and multiple pathologies. Due to considering of large image capacity we adapt Deep Convolutional Neural Network (DCNN) architecture for weakly-supervised object localization, different pooling strategies, various multi-label CNN losses and measured against a baseline of softmax regression.
Downloads
References
Wang X, Peng Y, Lu L, et al., IEEE Conference on “NIH clinical center provides one of the largest publicly available chest x-ray datasets toscientificcommunity,” pp. 2097-2106, Sep 2017.
E. Zimmermann, “Mayo clinic researchers’ demon- strate value of second opinions, “Journal of Evaluation of clinical pactice, https:// newsnetwork.mayoclinic.org/discussion/ mayo-clinic-researchers-demonstrate-value-of-second-opinions, April 2017.
D. Wang, A. Khosla, R. Gargeya, et al., “Deep learning for identifying metastatic breast cancer,” arXiv [q-bio.QM], June 2016.
M. Yann and Y. Tang, “Learning deep convolutional neural networks for X-ray protein crystallization image analysis,” Proc. Conf. AAAI Artif. Intell., vol. 30, no. 1, pp. 1373- 1379, Feb 2016.
P. Rajpurkar, J. Irvin, B. Yang, et al., “CheXNet: Radiologist-level pneumonia detection on chest X-rays with deep learning, “arXiv :1711.05225, vol.1, pp.1-7, Dec 2017.
V. Vryniotis “Machine learning tutorial:The logistic regression (softmax regression) “.,http://blog.datumbox.com/machine-learning-tutorial-the-multinomial-logistic-regression-softmax-regression, Nov 2013.
K. He, X. Zhang, S. Ren et al., “Deep residual learning for image recognition,” in 2016 IEEE Conference on Computer Vision and Pattern Recognition (CVPR), pp. 770-778, 2016.
M. Oquab, L. Bottou, I. Laptev, et al., “Is object localization for free? - Weakly-supervised learning with convolutional neural networks,” in 2015 IEEE Conference on Computer Vision and Pattern Recognition (CVPR), pp. 685–694 Jun 2015.
B. Zhou, A. Khosla, A. Lapedriza, et al., “Learning deep features for discriminative localization,”. arXiv:1512.04150 Dec 2015.
T. Durand, M. Cord and N. Thome Weldon: “Weakly supervised learning of deep convolutional neural networks, “Proceedings of IEEE Conference on Computer Vision and Pattern Recognition (CVPR), pp.4743-4752, June 2016
S .Hwang, H. E. Kim “Self-Transfer Learning for Weakly Supervised Lesion Localization, “In Medical Image Computing and Computer-Assisted Intervention – MICCAI, Lecture Notes in Computer Science. Springer, Cham, Vol 9901, no.2, pp.239– 246, Oct 2016.
J. Deng, W. Dong, R. Sochere et al., “ImageNet: A large-scale hierarchical image database,” in IEEE Conference on Computer Vision and Pattern Recognition, pp. 248-255, June 2009.
O. Russakovsky, J. Deng, H. Su, et al., “ImageNet large scale visual recognition challenge,” Int. J. Comput. Vis., vol. 115, no. 3, pp. 211–252, April 2015.
A. Krizhevsky, G. E. Hinton, and I. Sutskever, “Imagenet classification with deep convolutional neural networks, “In Advances in neural information processing systems, pp.1097–1105, 2012.
C. Szegedy, W. Lei,Y. Jia, et al., “Going deeper with convolutions,” in IEEE Conference on Computer Vision and Pattern Recognition (CVPR), pp. 1-9, June 2015.
K. Simonyan and A. Zisserman, “Very deep convolutional networks for large-scale image recognition,” arXiv:1409.1556, April 2014.
K. He, X. Zhang, S. Ren, et al., “Deep residual learning for image recognition,” in IEEE Conference on Computer Vision and Pattern Recognition (CVPR), arXiv preprint arXiv:1512.03385, Dec 2015.
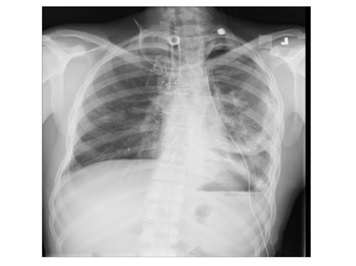