Multiclass Brain Tumor Classification Using Transfer Learning

DOI:
https://doi.org/10.54060/jieee.2024.95Keywords:
Magnetic Resonance Imaging, Deep Residual Network , glioma tumor, meningioma tumor, pituitary tumorAbstract
Tumors are a collection of abnormal cells that multiply enormously than required which leads to cancer and divergent and also can be fatal, if not identified at an early stage. Usually, brain scan described as Magnetic resonance imaging (MRI) is deployed for high transparency and representation in different angles but causes huge delay in declaring the result of the test. In this project, images obtained from these tests are carefully observed and classified by implementing Deep Residual Network (RESNET) to classify the type of tumor. There are four types of tumors such as glioma, meningioma, pituitary, and no tumor. Brain tumor classification (Multi Label) – CNN dataset has been imported to train and test the model. This deep learning model is a sophisticated approach which is developed to classify the tumor based on the image, so that appropriate treatment can be given on time. The output determines the type of tumor if present, otherwise no tumor with accuracy of 87% using epochs.
Downloads
References
A. Çinar and M. Yildirim, “Detection of tumors on brain MRI images using the hybrid convolutional neural network archi-tecture,” Med. Hypotheses, vol. 139, no. 109684, p. 109684, 2020.
M. Sajjad, S. Khan, K. Muhammad, W. Wu, A. Ullah, and S. W. Baik, "Multi-grade brain tumor classification using deep CNN with extensive data augmentation," Journal of computational science, vol. 30, pp. 174 – 182, 2019.
H. H. Sultan, N. M. Salem, and W. Al-Atabany, “Multi-classification of brain tumor images using deep neural net-work,” IEEE Access, vol. 7, pp. 69215–69225, 2019.
S. Hussain, S. M. Anwar, and M. Majid, "Segmentation of glioma tumors in brain using deep convolutional neural net-work," Neurocomputing, vol. 282, pp. 248-261, 2018.
P. Sharma, I. Wahlang, S. Sanyal, and A. K. Maji, “Classification of brain MRI using deep learning techniques,” in Advances in Intelligent Systems and Computing, Singapore: Springer Singapore, pp. 559–569, 2020.
A. Pundir and E. Rajeev Kumar, “Brain tumor classification in MRI images using transfer learning,” in Studies in Big Data, Singapore: Springer Singapore, pp. 307–319, 2021.
S. N. Noreen, A. Palaniappan, I. Qayyum, and M. O. Ahmad, “Brain Tumor Classification Based on Fine- Tuned Models and the Ensemble Method,” CMC-COMPUTERS MATERIALS & CONTINUA, vol. 67, no. 3, pp. 3967–3982, 2021.
Z. A. Sejuti and M. S. Islam, "An Efficient Method to Classify Brain Tumor using CNN and SVM," in 2021 2nd International Conference on Robotics, Electrical and Signal Processing Techniques (ICREST), pp. 644-648: IEEE, 2021.
Z. N. K. Swati et al., "Brain tumor classification for MR images using transfer learning and finetuning," Computerized Med-ical Imaging Graphics, vol. 75, pp. 34-46, 2019.
A. K. Anaraki, M. Ayati, and F. Kazemi, "Magnetic resonance imaging-based brain tumor grades classification and grading via convolutional neural networks and genetic algorithms," Biocybernetics Biomedical Engineering, vol. 39, no. 1, pp. 63-74, 2019.
M. Gurbină, M. Lascu and D. Lascu, "Tumor Detection and Classification of MRI Brain Image using Different Wavelet Transforms and Support Vector Machines," 2019 42nd International Conference on Telecommunications and Signal Pro-cessing (TSP), Budapest, Hungary, 2019, pp. 505-508, doi: 10.1109/TSP.2019.8769040.
H. T. Zaw, N. Maneerat, and K. Y. Win, “Brain tumor detection based on naive bayes classification,” in 5th International conference on engineering, applied sciences and technology (ICEAST), 2019, pp. 1–4.
S. A. Shimanto, M. K. Hosain, S. P. Biswas and M. S. Islam, "Brain Tumor Detection and Classification by SVM Algorithm and Performance Analysis Through CNN Approach," 2023 International Conference on Electrical, Computer and Commu-nication Engineering (ECCE), Chittagong, Bangladesh, 2023, pp. 1-6, doi: 10.1109/ECCE57851.2023.10101618.
J. Kakarla, B. V. Isunuri, K. S. Doppalapudi, and K. S. R. Bylapudi, “Three‐class classification of brain magnetic resonance images using average‐pooling convolutional neural network”. Int. J. Imaging Syst. Technol., vol. 31, no. 3, pp. 1731–1740, 2021.
T. L. Narayana and T. S. Reddy, "An Efficient Optimization Technique to Detect Brain Tumor from MRI Images," 2018 In-ternational Conference on Smart Systems and Inventive Technology (ICSSIT), Tirunelveli, India, 2018, pp. 168-171, doi: 10.1109/ICSSIT.2018.8748288.
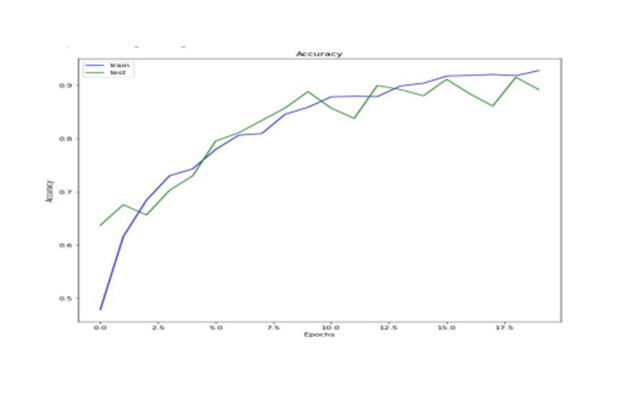
Downloads
Published
How to Cite
CITATION COUNT
License
Copyright (c) 2024 Dr. G. JayaLakshmi

This work is licensed under a Creative Commons Attribution 4.0 International License.