A Review on the Development of Fuzzy Classifiers with Improved Interpretability and Accuracy Parameters

DOI:
https://doi.org/10.54060/JIEEE/002.02.020Keywords:
Fuzzy logic, Fuzzy set, Crisp sets, Accuracy and Interpretability trade off, Type-2 fuzzy system, Interval type-2 fuzzy systemAbstract
This review paper of fuzzy classifiers with improved interpretability and accuracy param-eter discussed the most fundamental aspect of very effective and powerful tools in form of probabilistic reasoning, The fuzzy logic concept allows the effective realization of ap-proximate, vague, uncertain, dynamic, and more realistic conditions, which is closer to the actual physical world and human thinking. The fuzzy theory has the competency to catch the lack of preciseness of linguistic terms in a speech of natural language. The fuzzy theory provides a more significant competency to model humans like com-mon-sense reasoning and conclusion making to fuzzy set and rules as good membership function. Also, in this paper reviews discussed the evaluation of the fuzzy set, type-1, type-2, and interval type-2 fuzzy system from traditional Boolean crisp set logic along with interpretability and accuracy issues in the fuzzy system.
Downloads
References
L.A. Zadah, “Fuzzy sets”, Information and Control, vol. 8, No. 3, pp.338–353,1956.
J. F. Baldwin, “A new approach to approximate reasoning using a fuzzy logic,” Fuzzy Sets and Systems, vol. 2, no. 4, pp. 309–325, 1979.
J. F. Baldwin and N. C. F. Guild, “Feasible algorithms for approximate reasoning using fuzzy logic,” Fuzzy Sets and Systems, vol. 3, no. 3, pp. 225–251, 1980.
R. Czabański, J. Jeżewski, K. Horoba, and M. Jeżewski, “Fetal state assessment using fuzzy analysis of fetal heart rate signals—Agreement with the neonatal outcome,” Biocybern. Biomed. Eng., vol. 33, no. 3, pp. 145–155, 2013.
E.Czogala, J.Leski,”Fuzzy and Neuro-Fuzzy Intelligent Systems”. Physica-Verlag, SpringerComp., Heidelberg vol.47,2000.
E. Czogał a and J. Łęski, “On equivalence of approximate reasoning results using different interpretations of fuzzy if–then rules,” Fuzzy Sets and Systems, vol. 117, no. 2, pp. 279–296, 2001.
W. T. Dobrosielski, J. Szczepański, and H. Zarzycki, “A proposal for a method of defuzzification based on the golden ratio—GR,” in Advances in Intelligent Systems and Computing, Cham: Springer International Publishing, vol. 401,pp. 75–84,2016,
D.Dubois, & H.Prade,” Fuzzy sets in approximate reasoning - part 1: inference with possibilitydistributions” Fuzzy Sets Syst.vol. 40,pp. 143–202, 1991.
D. Dubois and H. Prade, “What are fuzzy rules and how to use them,” Fuzzy Sets and Systems, vol. 84, no. 2, pp. 169–185, 1996.
J. C. Fodor, “On fuzzy implication operators,” Fuzzy Sets and Systems, vol. 42, no. 3, pp. 293–300, 1991.
M.M.Ahmad, and N.A.M.Isa, “Knowledge base to fuzzy information granule: a review from the interpretability-accuracy perspective”, Applied Soft Computing, vol. 2017, pp.121–140 ,2017.
J.M.Alonso, and L.Magdalena,“An experimental study on the interpretability of fuzzy systems”, in Proceedings of International Fuzzy Systems Association World Congress andEuropean Society of Fuzzy Logic and Technology Conference (IFSA-EUSFLAT), pp.125–130 ,July 2009.
R. Hosseini, J. Dehmeshki, S. Barman, et al., "A Genetic type-2 fuzzy logic system for pattern recognition in computer aided detection systems," International Conference on Fuzzy Systems, Barcelona, Spain, pp. 1-7, 2010.
P. K. Shukla and S. P. Tripathi, "A Survey on Interpretability-Accuracy (I-A) Trade-Off in Evolutionary Fuzzy Systems," Fifth International Conference on Genetic and Evolutionary Computing, pp. 97-101,2011.
J. C. Bansal, P. K. Singh, K. Deep, et al., Proceedings of seventh international conference on bio-inspired computing: Theories and applications (BIC-TA ). India: Springer India, 2013.
P. K. Shukla and S. P. Tripathi, "On the Design of Interpretable Evolutionary Fuzzy Systems (I-EFS) with Improved Accuracy," International Conference on Computing Sciences, pp.11-14, 2012.
P. K. Shukla and S. P. Tripathi,"A review on the interpretability-accuracy trade-off in evolutionary multi-objective fuzzy systems (EMOFS)."Information vol.3,no.3 ,pp.256-277,2012.
P. K. Shukla and S. P. Tripathi, "A new approach for tuning interval type-2 fuzzy knowledge bases using genetic algorithms." Journal of Uncertainty Analysis and Applications vol.2,no.1 ,pp.1-15,2014.
P. K. Shukla and S. P. Tripathi, "Interpretability and accuracy issues in evolutionary multi-objective fuzzy classifiers." International Journal of Soft Computing and Networking vol.1,no.1, pp.55-69,2016.
P. K. Shukla, S. P. Tripathi, M.Darbari, et al. "A survey of fuzzy techniques in object-oriented data-bases." International Journal of Scientific and Engineering Research vol.2, no.11 ,pp.1-11,2011.
P. K. Shukla and S. P. Tripathi, " Uncertainty handling using fuzzy logic in rule based systems,“International Journal of Advanced Science and Technology,vol 45 pp. 31-46,2012.
P. K. Shukla and S. P. Tripathi, “A review on the Interpretability-Accuracy Trade-off in Evolutionary Multi-objective fuzzy systems (EMOFS),” Information (Basel), vol. 3, no. 3, pp. 256–277, 2012.
J. Casillas, O. Cordón, F. Herrera, et al.., Interpretability Issues in Fuzzy Modeling. Berlin, Heidelberg: Springer Berlin Heidelberg, 2003.
O. Castillo and P. Melin, “A review on the design and optimization of interval type-2 fuzzy controllers,” Appl. Soft Comput., vol. 12, no. 4, pp. 1267–1278, 2012.
O. Castillo and P. Melin, “A review on the design and optimization of interval type-2 fuzzy controllers”, Applied Soft Computing, vol. 12, no. 4, pp.1267–1278,2012.
Shukla, Praveen Kumar.” Development of Fuzzy Knowledge-Based System for Water Quality Assessment in River Ganga." Soft Computing for Problem Solving. Springer, Singapore, 2020. 17-26.
M.Topaloghu,F. Yarkin, and T.Kaya, “Solid waste collection system selection for smart cities based on a type-2 fuzzy multi criteria decision technique", Soft Computing, vol. 22, No. 15, pp.4879–4890,2018.
S.Tahilyani, M. Darbari, and P. K.Shukla, "Soft computing ap-proaches in traffic control systems: a review." Aasri Procedia vol.4 ,pp.206-211, 2013.
S.Tahilyani, M. Darbari, and P. K.Shukla, "A new genetic algorithm based lane-by-pass approach for smooth traffic flow on road networks." International Journal of Advanced Research in Artificial Intelligence, vol.1, no.3, pp.32-36, 2012.
A. Tiwari, V. K. Singh, and P. K. Shukla, “Mobile handset selection using evolutionary multi-objective optimization considering the cost and quality parameters,” in Communications in Computer and Information Science, Singapore: Springer Singapore, , pp. 259–268,2018.
A. Kaur and P. K. Shukla, "A review on evolutionary multiobjective optimization for routing problem of computer networks," Confluence , The Next Generation Information Technology Summit (4th International Conference), pp. 290-296,2013.
G.K.Shankhdhar,V K Singh and M Darbari, “Legal Semantic Web- A Recommendation System, “ International Journal of Applied Information Systems ,vol.7, no.3, pp.21-27, May 2014 .
P. Chandra, D. Agarwal, and P. K. Shukla, “MOBI-CLASS: A fuzzy knowledge-based system for mobile handset classification,” in Advances in Intelligent Systems and Computing, Singapore: Springer Singapore, , pp. 979–987,2019.
J. R. Castro, O. Castillo and P. Melin, "An Interval Type-2 Fuzzy Logic Toolbox for Control Applications," IEEE International Fuzzy Systems Conference, pp. 1-6 ,2007.
J. R. Castro, O. Castillo, P. Melin,et al., “A hybrid learning algorithm for a class of interval type-2 fuzzy neural networks,” Inf. Sci. (Ny), vol. 179, no. 13, pp. 2175–2193, 2009.
C. W. Lou and M. C. Dong, “A novel random fuzzy neural networks for tackling uncertainties of electric load forecasting,” Int. j. electr. power energy syst., vol. 73, pp. 34-44, 2015.
T.Chen, C. Shang, P.Su, et al., “Induction of accurate and interpretable fuzzy rules from preliminary crisp representation”, Knowledge Based System, vol. 146, pp.152–166, 2018.
R. Davoodi and M. H. Moradi, “Mortality prediction in intensive care units (ICUs) using a deep rule-based fuzzy classifier,” J. Biomed. Inform., vol. 79, pp. 48–59, 2018.
M. E.Bardini and A. M. E.Nagar, “Interval type-2 fuzzy PID controller for uncertain nonlinear inverted pendulum system,” ISA Trans., vol. 53, no. 3, pp. 732–743, 2014.
C. Fuchs, A. Wilbik and U. Kaymak, "Towards More Specific Estimation of Membership Functions for Data-Driven Fuzzy Inference Systems," IEEE International Conference on Fuzzy Systems (FUZZ-IEEE), pp. 1-8, 2018.
Y. Gao, F. Xiao, J. Liu et al., "Distributed Soft Fault Detection for Interval Type-2 Fuzzy-Model-Based Stochastic Systems With Wireless Sensor Networks," in IEEE Transactions on Industrial Informatics, vol. 15, no. 1, pp. 334-347, Jan. 2019.
M. B. Gorzałczany and F. Rudziński, "ECG Time Series Classification via Genetic-Fuzzy Approach Based on Accuracy-Interpretability Trade-Off Optimization," IEEE International Conference on Fuzzy Systems (FUZZ-IEEE), pp. 1-8, 2018,.
J. Huang, M. Ri, D. S. Ri,et al., "Interval Type-2 Fuzzy Logic Modeling and Control of a Mobile Two-Wheeled Inverted Pendulum," in IEEE Transactions on Fuzzy Systems, vol. 26, no. 4, pp. 2030-2038, Aug 2018.
Y. Jarraya, S. Bouaziz, H. Hagras, et al., “A multi-agent architecture for the design of hierarchical interval type-2 beta fuzzy system,” IEEE Trans. Fuzzy Syst., vol. 27, no. 6, pp. 1174–1188, 2019.
N.N.Karnik, and J.M.Mendel, “Centroid of type-2 fuzzy set”, Information Sciences, vol. 132, no.1, pp.195–220 2001.
K. Łapa, K. Cpałka, and L. Rutkowski, “New aspects of interpretability of fuzzy systems for nonlinear modeling,” in Advances in Data Analysis with Computational Intelligence Methods, Cham: Springer International Publishing, vol.738,pp. 225–264,2018
F.Olivas, F.Valdez, O.Castillo, et al., “Ant colony optimization with dynamic parameter adaptation based on interval type-2 fuzzy logic systems”, Applied Soft Computing, April, vol. 53, pp.74–87,2017.
J.Perez, F.Valdez, O. Castillo, et al., “Interval type-2 fuzzy logic for dynamic parameter adaptation in the Bat algorithm”, Soft Computing, vol. 21, no. 3, pp.667–685,2017.
M.Pota, M.Esposito, and G.D. Pietro, “Likelihood-fuzzy analysis: from data, through statistics, to interpretable fuzzy classifiers”, International Journal of Approximate Reasoning, vol. 93, pp.88–102 ,2018.
S,Rajab, and V.Sharma, “An interpretable neuro fuzzy approach to stock price forecasting”, Soft Computing, vol. 28, pp.1–16, 2017.
T. R. Razak, J. M. Garibaldi, C. Wagner, et al., "Interpretability and Complexity of Design in the Creation of Fuzzy Logic Systems — A User Study," 2018 IEEE Symposium Series on Computational Intelligence (SSCI), pp. 420-426, 2018.
M. I. Rey, M. Galende, M. J. Fuente, et al., “Multi-objective based Fuzzy Rule Based Systems (FRBSs) for trade-off improvement in accuracy and interpretability: A rule relevance point of view,” Knowl. Based Syst., vol. 127, pp. 67–84, 2017.
M. Ricatto, M. Barsacchi, and A. Bechini, “Interpretable CNV-based tumour classification using fuzzy rule based classifiers,” in Proceedings of the 33rd Annual ACM Symposium on Applied Computing, pp.54–59, 2018.
H. Roubos and M. Setnes, "Compact and transparent fuzzy models and classifiers through iterative complexity reduction," in IEEE Transactions on Fuzzy Systems, vol. 9, no. 4, pp. 516-524, Aug. 2001 .
Shalaginov,& Andrii. "Fuzzy logic model for digital forensics: A trade-off between accuracy, complexity and interpretability." IJCAI International Joint Conference on Artificial Intelligence, pp.5207–5208,2017.
P. A. Herman, G. Prasad and T. M. M. Ginnity, "Designing an Interval Type-2 Fuzzy Logic System for Handling Uncertainty Effects in Brain–Computer Interface Classification of Motor Imagery Induced EEG Patterns," in IEEE Transactions on Fuzzy Systems, vol. 25, no. 1, pp. 29-42, Feb. 2017.
D. Yagyasen, M. Darbari, P. K. Shukla, et al., “Diversity and convergence issues in evolutionary multiobjective optimization: Application to agriculture science,” IERI Procedia, vol. 5, pp. 81–86, 2013.
R. Asthana, N. J.Ahuja, M.Darbari, et al., "A critical review on the development of urban traffic models & control systems," Int. J. Sci. Eng. Res vol.3.no.1,pp.1-6,2012.
S. R. M. M. Roveda, A. P. M. Bondança, J. G. S. Silva,et al., "Development of a water quality index using a fuzzy logic: A case study for the Sorocaba river," International Conference on Fuzzy Systems, pp. 1-5 ,2010,.
D. Scannapieco, V. Naddeo, T. Zarra, et al., “River water quality assessment: A comparison of binary- and fuzzy logic-based approaches,” Ecol. Eng., vol. 47, pp. 132–140, 2012.
L. M. Fleuren,Klaush,T.L.T,Zwgaer et al., “Machine learning for the prediction of sepsis: a systematic review and meta-analysis of diagnostic test accuracy,” Intensive Care Med., vol. 46, no. 3, pp. 383–400, 2020.
Qilian Liang and J. M. Mendel, "Interval type-2 fuzzy logic systems: theory and design," in IEEE Transactions on Fuzzy Systems, vol. 8, no. 5, pp. 535-550, Oct. 2000.
J. M. Mendel and F. Liu, "Super-Exponential Convergence of the Karnik–Mendel Algorithms for Computing the Centroid of an Interval Type-2 Fuzzy Set," in IEEE Transactions on Fuzzy Systems, vol. 15, no. 2, pp. 309-320, April 2007.
J. M. Mendel and F. Liu, "Super-Exponential Convergence of the Karnik–Mendel Algorithms for Computing the Centroid of an Interval Type-2 Fuzzy Set," in IEEE Transactions on Fuzzy Systems, vol. 15, no. 2, pp. 309-320, April 2007.
T. Rutkowski, J. Romanowski, P. Woldan, et al., “Towards interpretability of the movie recommender based on a neuro-fuzzy approach,” in Artificial Intelligence and Soft Computing, Cham: Springer International Publishing, pp. 752–762, 2018,
B. Wu, T. L. Yip, X. Yan, et al., “Fuzzy logic based approach for ship-bridge collision alert system,” Ocean Eng., vol. 187, no. 106152, 2019.
S. Kambalimath and P. C. Deka, “A basic review of fuzzy logic applications in hydrology and water resources,” Appl. Water Sci., vol. 10, no. 8, 2020.
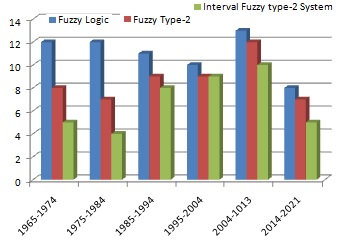