Characterizing and Predicting Reviews for Effective Product Marketing and Advancement

DOI:
https://doi.org/10.54060/JIEEE/002.02.006Keywords:
Online Review, Big data, Analysis, machine LearningAbstract
In the present made world, dependably, individuals around the planet grant through different stages on the Web. It has been addressed, about 71% of by and large online customers read online surveys going before buying a thing. Thing considers, particularly the early surveys (i.e., the investigations posted at the beginning time of a thing), astoundingly impact coming about thing deals. We call the clients who posted the early examinations as "early investigators". Be that as it may, early specialists contribute just a little level of surveys, their feelings can pick the achievement or disappointment of new things and associations. It is immense for relationship to perceive early spectators since their responses can assist relationship with changing publicizing frameworks and improve thing plans, which can at last incite the accomplishment of their new things. And in dependably, a mass extent of unstructured information is made. This information is as text, which is accumulated from get-togethers, online media regions, surveys. Such information is named as gigantic information. Client feelings are identified with a wide degree of spotlights like on express things also. These investigations can be mined utilizing different movements and are of everything considered significance to make checks since they unmistakably pass on the perspective of the bigger part. Online outlines moreover have become a basic wellspring of data for clients going before settling on an educated buy choice. Early examiner's appraisals and their got strength scores are apparently going to influence thing notoriety. The test is to assemble all the audits, in like way find and investigate the assessments, to locate something refined, that scores high evaluating.
Downloads
References
N. Sultana, P. Kumar, M.R. Patra, et al." Sentiment Analysis for Product Review" ICTACT Journal on Soft Computing, vol.9, no.03, April 2019.
A. Patel, and A.K. Tiwari,” Sentiment Analysis by using Recurrent Neural Network Proceedings of 2nd International Conference on Advanced Computing and Software Engineering (ICACSE) , pp.108-111, Feb 2019.
Y.-J. Park, “Predicting the helpfulness of online customer reviews across different product types,” Sustainability, vol. 10, no. 6, p. 1735, May 2018.
S. Saumya, J. Prakash Singh, N. P. Rana, et al., "Situating Online Consumer Reviews" Article in Electronic Commerce Research and Applications, March 2018.
S. Liao, J. Wang, R. Yu, et al., “CNN for situations understanding based on sentiment analysis of twitter data,” Procedia Comput. Sci., vol. 111, pp. 376–381, 2017.
X. Fang and J. Zhan, “Sentiment analysis using product review data,” J. Big Data, vol. 2, no. 1, June 2015.
D. Imamori and K. Tajima, "Foreseeing notoriety of twitter accounts through the revelation of link-propagating early adopters," in CoRR, p. 15-12, 2015.
A. V. Yeole, P. V. Chavan, and M. C. Nikose, “Opinion mining for emotions determination,” in International Conference on Innovations in Information, Embedded and Communication Systems (ICIIECS), 2015.
F. Luo, C. Li, and Z. Cao, “Affective-feature-based sentiment analysis using SVM classifier,” in IEEE 20th International Conference on Computer Supported Cooperative Work in Design (CSCWD), pp.276-281, Sep 2016.
Q.T.Ain , M.Ali, A.Riaz, et al. "Sentiment analysis using deep learning techniques: a review." International Journal of Advanced Computer Science and Applications, vol.8, no.6, 2017.
B. Liu, E. Blasch, Y. Chen, et al., “Scalable sentiment classification for Big Data analysis using Naïve Bayes Classifier,” in IEEE International Conference on Big Data, Dec 2013.
D. V. N. Devi, C. K. Kumar, and S. Prasad, “A feature-based approach for sentiment analysis by using support vector machine,” in IEEE 6th International Conference on Advanced Computing (IACC), pp.3-8, Aug 2016.
J. M.Auley and J. Leskovec, “Hidden factors and hidden topics: Understanding rating dimensions with review text,” in Proceedings of the 7th ACM conference on Recommender systems, pp.165-172,Oct 2013.
A. Ghose and P. G. Ipeirotis, “Estimating the helpfulness and economic impact of product reviews: Mining text and reviewer characteristics,” IEEE Trans. Knowl. Data Eng., vol. 23, no. 10, pp. 1498–1512, Oct 2011.
P. Singhal, P. Sharma, & S. Rizvi, “Thwarting Sybil Attack by CAM Method in WSN using Cooja Simulator Framework,” International Journal of Engineering & Technology, vol.8, no.1.5, pp.116-125, 2019.
M. Vinny, & P. Singh,” Review on the Artificial Brain Technology: BlueBrain,” Journal of Informatics Electrical and Electronics Engineering, vol.1, no.1, pp. 1-11,2020.
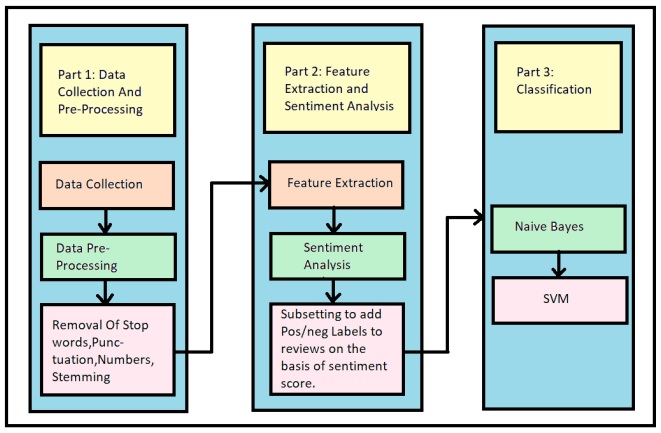