An Intelligent Particle Filter with Neural Network for Fault Location and Classification in Microgrid

DOI:
https://doi.org/10.54060/a2zjournals.jieee.128Keywords:
Microgrid, Fault detection, Fault location, particle filter estimation, neural networkAbstract
Microgrid concept is initiated due to increasing involvement of distributed generation resources with the utility grid. Microgrid provide reliable and sustainable power but the protection of microgrid become challenging due to bidirectional power flow, dual mode of operation (grid connected and islanded mode). Faults in the microgrid reduce its stability and efficiency. Identification, classification, and location of faults are crit-ical for rapid restoration and microgrid protection. This research proposes a neural network-based intelligent particle filter for microgrid fault detection and classifica-tion. Even with low fault current, which is typical of inverter-based DGs, the suggest-ed method seeks to precisely identify fault kinds, locations, and directions. The fea-tures are extracted from data using S-Transform, then extracted features are esti-mated using particle filter. A neural network is then used for classification and finali-zation of location. The proposed scheme provides extremely precise fault detection, ensuring that the classification and location of the fault are promptly identified for effective protection and service restoration.
Downloads
References
M. W. Altaf, M. T. Arif, S. N. Islam, M. E. Haque, “Microgrid Protection Challenges and Mitigation Approaches-A Comprehensive Review”, IEEE Access, 2022, doi: 10.1109/ACCESS.2022.3165011.
S. S. Rath, G. Panda, P. K. Ray, A. Mohanty, “A Comprehensive Review on Microgrid Protection: Issues and Challenges”, Proc. 3rd Int. Conf. on Energy, Power and Environment (ICEPE), pp. –, 2021, doi: 10.1109/ICEPE50861.2021.9404520.
M. A. Zamani, T. S. Sidhu, A. Yazdani, “A communication-based strategy for protection of microgrids with looped configuration”, Electric Power Systems Research, Vol.104, pp.52–61, 2013, doi: 10.1016/j.epsr.2013.06.006.
C. Chandraratne, T. N. Ramasamy, T. Logenthiran, G. Panda, “Adaptive protection for microgrid with distributed energy resources”, Electronics (Switzerland), Vol.9, No.11, pp.1–14, 2020, doi: 10.3390/electronics9111959.
M. A. Jarrahi, H. Samet, T. Ghanbari, “Novel Change Detection and Fault Classification Scheme for AC Microgrids”, IEEE Systems Journal, Vol.14, No.3, pp.3987–3998, 2020, doi: 10.1109/JSYST.2020.2966686.
E. Casagrande, W. L. Woon, H. H. Zeineldin, N. H. Kan’an, “Data mining approach to fault detection for isolated inverter-based microgrids”, IET Generation, Transmission and Distribution, Vol.7, No.7, pp.745–754, 2013, doi: 10.1049/iet-gtd.2012.0518.
S. N. V. Bramareswara Rao, Y. V. P. Kumar, M. Amir, S. M. Muyeen, “Fault detection and classification in hybrid energy-based multi-area grid-connected microgrid clusters using discrete wavelet transform with deep neural networks”, Electrical Engineering, 2024, doi: 10.1007/s00202-024-02329-4.
M. Mishra, P. K. Rout, “Detection and classification of micro-grid faults based on HHT and machine learning techniques”, IET Generation, Transmission and Distribution, Vol.12, No.2, pp.388–397, 2018, doi: 10.1049/iet-gtd.2017.0502.
J. A. R. R. Jayasinghe, J. H. E. Malindi, R. M. A. M. Rajapaksha, V. Logeeshan, C. Wanigasekara, “Classification and Localization of Faults in AC Microgrids Through Discrete Wavelet Transform and Artificial Neural Networks”, IEEE Open Access Journal of Power and Energy, Vol.11, pp.303–313, 2024, doi: 10.1109/OAJPE.2024.3422387.
F. Mumtaz et al., “A Kalman Filter-Based Protection Strategy for Microgrids”, IEEE Access, Vol.10, pp.73243–73256, 2022, doi: 10.1109/ACCESS.2022.3190078.
N. Sapountzoglou, J. Lago, B. Raison, “Fault diagnosis in low voltage smart distribution grids using gradient boosting trees”, Electric Power Systems Research, Vol.182, 2020, doi: 10.1016/j.epsr.2020.106254.
S. Baloch, M. S. Muhammad, “An Intelligent Data Mining-Based Fault Detection and Classification Strategy for Microgrid”, IEEE Access, Vol.9, pp.22470–22479, 2021, doi: 10.1109/ACCESS.2021.3056534.
S. B. A. Bukhari, C. H. Kim, K. K. Mehmood, R. Haider, M. S. U. Zaman, “Convolutional neural network-based intelligent protection strategy for microgrids”, IET Generation, Transmission and Distribution, Vol.14, No.7, pp.1177–1185, 2020, doi: 10.1049/iet-gtd.2018.7049.
D. Liu, A. Dy, Q. Hong, S. Member, D. Tzelepis, C. Booth, “Transient Wavelet Energy Based Protection Scheme for Inverter-Dominated Microgrid”, unpublished.
F. Mumtaz et al., “High Impedance Faults Detection and Classification in Renewable Energy-Based Distribution Networks Using Time-Varying Kalman Filtering Technique †”, Engineering Proceedings, Vol.20, No.1, 2022, doi: 10.3390/engproc2022020034.
X. Zheng, Y. Zeng, M. Zhao, B. Venkatesh, “Early Identification and Location of Short-Circuit Fault in Grid-Connected AC Microgrid”, IEEE Transactions on Smart Grid, Vol.12, No.4, pp.2869–2878, 2021, doi: 10.1109/TSG.2021.3066803.
O. A. Gashteroodkhani, M. Majidi, M. S. Fadali, M. Etezadi-Amoli, E. Maali-Amiri, “A Protection Scheme for Microgrids Using Time-Time Matrix Z-score Vector”, 2019. Available: https://www.elsevier.com/open-access/userlicense/1.0/
N. Giri et al., “Wavelet-Based ensembled intelligent technique for advanced fault detection and classification in AC microgrids”, Energy Conversion and Management: X, Vol.24, 2024, doi: 10.1016/j.ecmx.2024.100813.
D. Liu, S. Wang, W. Su, X. Zhang, S. Hui, “Protection and security method for multiple energy power plant-based microgrids using dual filtering algorithm”, IEEE Access, 2024, doi: 10.1109/ACCESS.2024.3522556.
C. Cepeda et al., “Intelligent fault detection system for microgrids”, Energies (Basel), Vol.13, No.5, 2020, doi: 10.3390/en13051223.
A. R. Aqamohammadi, T. Niknam, S. Shojaeiyan, P. Siano, M. Dehghani, “Deep Neural Network with Hilbert–Huang Transform for Smart Fault Detection in Microgrid”, Electronics (Switzerland), Vol.12, No.3, 2023, doi: 10.3390/electronics12030499.
S. Kar, S. R. Samantaray, M. D. Zadeh, “Data-Mining Model Based Intelligent Differential Microgrid Protection Scheme”, IEEE Systems Journal, Vol.11, No.2, pp.1161–1169, 2017, doi: 10.1109/JSYST.2014.2380432.
S. R. Fahim, S. K. Sarker, S. M. Muyeen, M. R. I. Sheikh, S. K. Das, “Microgrid fault detection and classification: Machine learning based approach, comparison, and reviews”, Energies (Basel), Vol.13, No.13, 2020, doi: 10.3390/en13133460.
IEEE Power & Energy Society General Meeting (PESGM), 4-8 August 2019, IEEE, 2019.
M. Shafiullah, M. A. Abido, “S-Transform Based FFNN Approach for Distribution Grids Fault Detection and Classification”, IEEE Access, Vol.6, pp.8080–8088, 2018, doi: 10.1109/ACCESS.2018.2809045.
D. Li, A. Ukil, K. Satpathi, Y. M. Yeap, “Improved S Transform-Based Fault Detection Method in Voltage Source Converter Interfaced DC System”, IEEE Transactions on Industrial Electronics, Vol.68, No.6, pp.5024–5035, 2021, doi: 10.1109/TIE.2020.2988193.
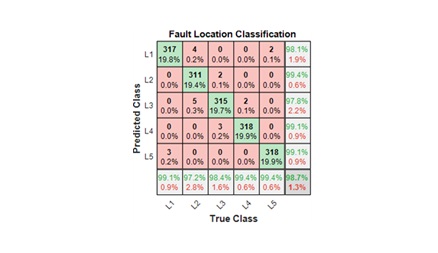
Downloads
Published
How to Cite
CITATION COUNT
License
Copyright (c) 2025 Archana, S.K. Sharma

This work is licensed under a Creative Commons Attribution 4.0 International License.