An In-Depth Evaluation of Recommendation Systems: Methods, Challenges, and Solutions

DOI:
https://doi.org/10.54060/a2zjournals.jieee.111Keywords:
Recommendation systems, content-based filtering, collaborative filtering, hybrid recommendation systems, cold-start problemAbstract
Recommendation systems (RS) play a vital role in the digital landscape, in shaping user experiences across various platforms. It delves into the origins and key characteristics of Content-Based Filtering and Collaborative Filtering, backed by empirical analysis to underscore their practical significance. It will go through the intricate development stages of RS, spanning from data investigation to prediction methodologies, and tackles challenges such as the cold-start problem. RS is categorized into three main types: collaborative filtering, content-based filtering, and hybrid recommendation systems, highlighting their potential synergy in enhancing recommendation accuracy result and breadth. These insights lay the groundwork for subsequent, which explore evaluation techniques, seminal research, dataset analysis, and experimental findings, concluding with reflections and avenues for future research to advance the field of recommendation systems.
Downloads
References
V. M, and T. K, “History and overview of the recommender systems”, in Collaborative Filtering Using Data Mining and Analysis, V. Bhatnagar, Ed. Hershey: IGI Global, pp. 74-99, 2016.
M. Rouse, “What is collaborative filtering?”, WhatIs.com, para. 1, Aug. 11, 2017, [Online], Available: https://whatis.techtarget.com/definition/collaborative-filtering
C.S. Perone, “Machine Learning :: Cosine Similarity for Vector Space Models (Part III)”, Dec. 09, 2013, [Online], Available: http://blog.christianperone.com/2013/09/machine-learning-cosine-similarity-for-vector- space-models-part-iii/
B. Sarwar, G. Karypis, J. Konstan and R. John, “Item-based Collaborative Filtering recommendation algorithms”, pp. 285-295, 2001.
Z. Zhao, M. Shang, “User-based collaborative-filtering recommendation algorithms on Hadoop”, in Third International Conference on Knowledge Discovery and Data Mining, Phuket, Thailand, IEEE, pp. 478-481, 2010.
R. V. Meteren, M. V. Someren, “Using Content-Based Filtering for recommendation”, 2000.
Y. Shih and D. Liu, “Hybrid recommendation approaches: Collaborative Filtering via valuable content information”, in Proceedings of the 38th Annual Hawaii International Conference on System Sciences, Big Island, USA, IEEE, pp. 217b-217b, 2005.
G. Adomavicius and A. Tuzhilin, “Toward the next generation of recommender systems: A survey of the state-of-the-art and possible extensions”, in IEEE Transactions on Knowledge & Data Engineering, pp. 734-749, 2005.
C. Ziegler, S. M. McNee, J. A. Konstan and G. Lausen, “Improving recommendation lists through topic diversification”, in Proceedings of the 14th international conference on World Wide Web, Chiba, Japan, ACM, pp. 22-32, 2005.
S. Min and I. Han, “Detection of the customer time-variant pattern for improving recommender systems”, Expert systems with applications, vol. 28, no. 2, pp. 189-199., Nov. 2004
H. Lieberman, “Letizia: An Agent That Assists Web Browsing”, IJCAI, pp. 924-929, 1995.
B. Mobasher, “Recommender Systems,” Kunstliche Intelligenz, Special Issue on Web Mining, BottcherIT Verlag, Bremen, Germany, pp. 41-43, 2007.
M. Göksedef and S. G. Öğüdücü, “Combination of Web page recommender systems”, Expert systems with applications, vol. 37, no. 4, pp. 2911-2922., Apr 2010.
M. Y. H. Al-Shamri and K. K. Bharadwaj, “Fuzzy-genetic approach to recommender systems based on a novel hybrid user model”, Expert systems with applications, vol. 35, no. 3, pp.1386-1399., Oct. 2008.
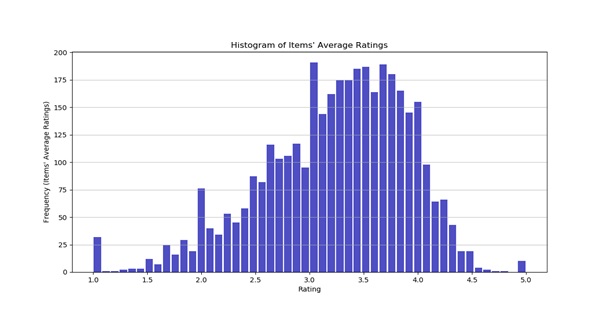
Downloads
Published
How to Cite
CITATION COUNT
License
Copyright (c) 2020 Pranjal Kumar Singh, Vineet Singh, Shikha Singh, Bramah Hazela

This work is licensed under a Creative Commons Attribution 4.0 International License.