A Study on Opinion Spamming: Fake Consumer Review Detection

DOI:
https://doi.org/10.54060/JIEEE/002.02.004Keywords:
Spam, Big data, machine learning, detectionAbstract
Online audits are the most important wellsprings of data about client feelings and are considered the columns on which the standing of an association is assembled. From a client's viewpoint, audit data is vital to settle on an appropriate choice with respect to an online buy. Surveys are for the most part thought to be a fairminded assessment of a person's very own involvement in an item, however, the fundamental truth about these audits recounts an alternate story. Spammers abuse these audit stages unlawfully on account of impetuses engaged with composing counterfeit surveys, subsequently attempting to acquire a bit of leeway over contenders bringing about an unstable development of assessment spamming. This training is known as Opinion Spam, where spammers control and toxic substance surveys for benefit or gain. In the event that one sees numerous positive audits of the item, one is probably going to purchase the item. Notwithstanding, in the event that one sees many negative surveys, he/she will in all probability pick another item. Positive suppositions can bring about huge monetary benefits and additionally popularities for associations and people. This, sadly, offers great motivating forces for input spam. Most of the momentum research has zeroed in on regulated learning strategies, which require named information, a shortage with regards to online survey spam. Examination of techniques for Big Data is of revenue, since there are a huge number of online audits, with a lot seriously being produced every day. Until now, we have not discovered any papers that review the impacts of Big Data examination for survey spam identification. The essential objective of this paper is to give a solid and farreaching similar investigation of flow research on identifying audit spam utilizing different AI procedures and to devise a strategy for directing further examination.
Downloads
References
E. I. Elmurngi and A. Gherbi, "Unreasonable Reviews Detection on Amazon Reviews utilizing Sentiment Analysis with Supervised Learning Techniques," Journal of Computer Science, vol. 14, no. 5, pp. 714–726, June 2018.
J. C. S. Reis, A. Correia, F. Murai, A. Veloso, and F. Benevenuto, “Supervised learning for fake news detection,” IEEE Intell. Syst., vol. 34, no. 2, pp. 76–81, May 2019.
B. Wagh, J. V. Shinde, and P. A. Kale, “A Twitter Sentiment Analysis using NLTK and machine learning techniques,” Int. j. emerg. res. manag. technol., vol. 6, no. 12, June 2018.
B. Liu, “Opinion Spam Detection,” in Sentiment Analysis and Opinion Mining, Cham: Springer International Publishing, pp. 113–125, 2012.
Y. Yao, B. Viswanath, J. Cryan, et al., “Automated crowdturfing attacks and defenses in online review systems,” in Proceedings of the ACM SIGSAC Conference on Computer and Communications Security, Oct 2017.
M. Ott, Y. Choi, C. Cardie, et al., “Finding deceptive opinion spam by any stretch of the imagination,” pp. 309–319, July 2011.
H. Li, G. Fei, S. Wang, et al., “Bimodal distribution and co-bursting in review spam detection,” in Proceedings of the 26th International Conference on World Wide Web, pp.1063-1072, April 2017.
R. Verma, and P. Hridayalankar,"Overview PAPER ON DETECTING FAKE SELLERS USING REVIEWS "Procedures of IEEE forum International Conference, pp.5-9, Dec 2019.
D. Martens and W. Maalej, “Towards understanding and detecting fake reviews in app stores,” Empir. Softw. Eng., vol. 24, no. 6, pp. 3316–3355, Dec 2019.
X. Wang, X. Zhang, C. Jiang et al., "Distinguishing proof of phony surveys utilizing semantic and social highlights," in fourth International Conference on Information Management (ICIM), Oxford, pp. 92-97,2018.
N. A. Patel and R. Patel, “A survey on fake review detection using machine learning techniques,” in 4th International Conference on Computing Communication and Automation (ICCCA), pp. 1-6, Dec 2018.
N. S. Chowdhary and A. A. Pandit. N. S. and A. A., “Fake Review Detection using Classification,” Int. J. Comput. Appl., vol. 180, no. 50, pp. 16–21, June 2018.
W. Liu, J. He, S. Han, et al., “A method for the detection of fake reviews based on temporal features of reviews and comments,” IEEE Eng. Manag. Rev., vol. 47, no. 4, pp. 67–79, July 2019.
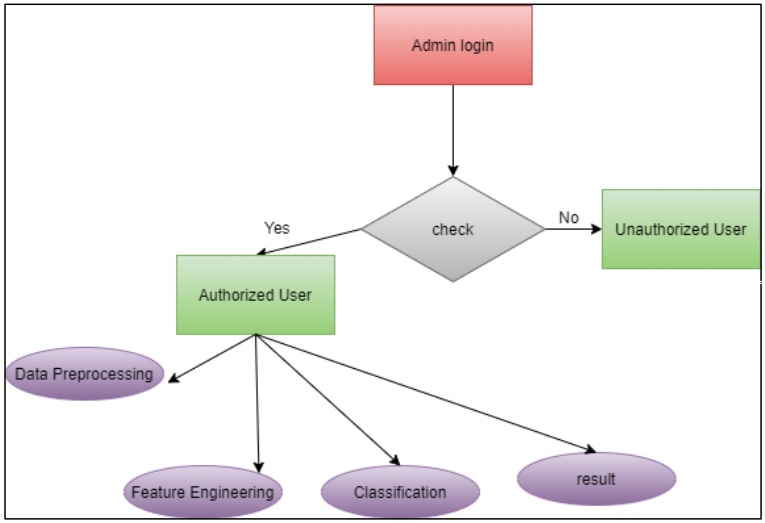