Multilevel SVM and AI based Transformer Fault Diagnosis using the DGA Data

DOI:
https://doi.org/10.54060/JIEEE/002.03.001Keywords:
Dissolved gas analysis, multilevel Support vector machine, Kernel Functions, Transformer fault diagnosis, Combination of ratios and graphical representationAbstract
The Dissolved Gas Analysis (DGA) is utilized as a test for the detection of incipient prob-lems in transformers, and condition monitoring of transformers using software-based diagnosis tools has become crucial. This research uses dissolved gas analysis as an intel-ligent fault classification of a transformer. The Multilayer SVM technique is used to de-termine the classification of faults and the name of the gas. The learned classifier in the multilayer SVM is trained with the training samples and can classify the state as normal or fault state, which contains six fault categories. In this paper, polynomial and Gaussi-an functions are utilized to assess the effectiveness of SVM diagnosis. The results demonstrate that the combination ratios and graphical representation technique is more suitable as a gas signature, and that the SVM with the Gaussian function outperforms the other kernel functions in diagnosis accuracy.
Downloads
References
S.-W. Fei and X.-B. Zhang, “Fault diagnosis of power transformer based on support vector machine with genetic algorithm,” Expert Syst. Appl., vol. 36, no. 8, pp. 11352–11357, 2009.
A. Akbari, A. Setayeshmehr, H. Borsi, E. Gockenbach, and I. Fofana, “Intelligentagent based system using dissolved gas analysis to detect incipient faults in power transformers,” IEEE Electrical Insulation Magazine, vol. 26, no. 6, pp. 27–40, 2010.
A. Shintemirov, W. Tang, and Q. H. Wu, “Power transformer fault classification based on dissolved gas analysis by implementing bootstrap and genetic programming,” IEEE Trans. Syst. Man Cybern. C Appl. Rev., vol. 39, no. 1, pp. 69–79, 2009.
Y.-J. Sun, S. Zhang, C.-X. Miao, and J.-M. Li, “Improved BP neural network for transformer fault diagnosis,” J. China Univ. Min. Technol. (Eng. Ed.), vol. 17, no. 1, pp. 138–142, 2007.
Z. J. Richardson, J. Fitch, W. H. Tang, J. Y. Goulermas, and Q. H. Wu, “A probabilistic classifier for transformer dissolved gas analysis with a particle swarm optimizer,” IEEE trans. power deliv., vol. 23, no. 2, pp. 751–759, 2008.
C.-P. Hung and M.-H. Wang, “Diagnosis of incipient faults in power transformers using CMAC neural network approach,” Electric Power Syst. Res., vol. 71, no. 3, pp. 235–244, 2004.
M. Duval, "A review of faults detectable by gas-in-oil analysis in transformers," in IEEE Electrical Insulation Magazine, vol. 18, no. 3, pp. 8-17, May-June 2002, doi: 10.1109/MEI.2002.1014963..
R. Hooshmand, M. Banejad, “Application of fuzzy logic in fault diagnosis in transformers using dissolved gas based on different standards,” World Academy of Science, Engineering and Technology, 17,pp.157–161,2006.
V. Miranda and A. R. G. Castro, “Improving the IEC table for transformer failure diagnosis with knowledge extraction from neural networks,” IEEE trans. power deliv., vol. 20, no. 4, pp. 2509–2516, 2005.
S.-W. Fei, C.-L. Liu, and Y.-B. Miao, “Support vector machine with genetic algorithm for forecasting of key-gas ratios in oil-immersed transformer,” Expert Syst. Appl., vol. 36, no. 3, pp. 6326–6331, 2009.
Standard IEC 60599, Guide for the interpretation of dissolved gas analysis and gas-free, 2007.
R.N. Afiqah, I. Musirin, D. Johari, M.M. Othman, T.K.A. Rahman, Z. Othman, Fuzzy logic application in DGA methods to classify fault type in power transformer, Selected Topics in Power Systems and Remote Sensing, Malaysia ,2008.
W. C. Flores, E. E. Mombello, J. A. Jardini, G. Rattá, and A. M. Corvo, “Expert system for the assessment of power transformer insulation condition based on type-2 fuzzy logic systems,” Expert Syst. Appl., vol. 38, no. 7, pp. 8119–8127, 2011.
R. Ziani, A. Felkaoui, R. Zegadi, Performances de la classification par les Séparateurs à Vaste Marge (SVM): application au diagnostic vibratoire automatisé, In the Proceedings of the 4th International Conference on Computer Integrated Manufacturing CIP’2007, 03–04 November, 2007.
L. V. Ganyun, C. Haozhong, Z. Haibao, and D. Lixin, “Fault diagnosis of power transformer based on multi-layer SVM classifier,” Electric Power Syst. Res., vol. 74, no. 1, pp. 1–7, 2005.
V.N. Vapnik, The Nature of Statistical Learning Theory, Springer-Verlag, New York, 1995.
W.-M. Lin, C.-H. Lin, and M.-X. Tasy, “Transformer-fault diagnosis by integrating field data and standard codes with training enhancible adaptive probabilistic network,” IEE Proc. - Gener. Transm. Distrib., vol. 152, no. 3, p. 335, 2005.
S. Ghosh and S. Dutta, “Ensemble machine learning methods for better dynamic assessment of transformer status,” J. Inst. Eng. (India) Ser. B, vol. 102, no. 5, pp. 1113–1122, 2021., https://doi.org/10.1007/s40031-021-00599-1.
B. Zeng et al., “Prediction model for dissolved gas concentration in transformer oil based on modified grey wolf optimizer and LSSVM with grey relational analysis and empirical mode decomposition,” Energies, vol. 13, no. 2, p. 422, 2020, https://doi:10.3390/en13020422.
R. Rogers, “IEEE and IEC codes to interpret incipient faults in transformers, using gas in oil analysis,” IEEE trans. electr. insul., vol. EI-13, no. 5, pp. 349–354, 1978, https://doi.org/10.1109/TEI.1978.298141.
A.-M. Aciu, C.-I. Nicola, M. Nicola, and M.-C. Nițu, “Complementary analysis for DGA based on Duval methods and furan compounds using artificial neural networks,” Energies, vol. 14, no. 3, p. 588, 2021. https://doi.org/10.3390/en14030588.
E. T. Mharakurwa, G. N. Nyakoe, and A. O. Akumu, “Power transformer fault severity estimation based on dissolved gas analysis and energy of fault formation technique,” J. Electr. Comput. Eng., vol. 2019, pp. 1–10, 2019. https://doi.org/10.1155/2019/9674054.
A. Abu-Siada, “Improved consistent interpretation approach of fault type within power transformers using dissolved gas analysis and gene Expression Programming,” Energies, vol. 12, no. 4, p. 730, 2019. https://doi.org/10.3390/en12040730.
N. Poonnoy, C. Suwanasri, and T. Suwanasri, “Fuzzy logic approach to dissolved gas analysis for power transformer failure index and fault identification,” Energies, vol. 14, no. 1, p. 36, 2020. https://dx.doi.org/10.3390/en14010036.
J. Faiz and M. Soleimani, “Dissolved gas analysis evaluation in electric power transformers using conventional methods a review,” IEEE Trans. Dielectr. Electr. Insul., vol. 24, no. 2, pp. 1239–1248, 2017, https://dx.doi.org/ 10.1109/TDEI.2017.005959.
J. Faiz and M. Soleimani, “Assessment of computational intelligence and conventional dissolved gas analysis methods for transformer fault diagnosis,” IEEE Trans. Dielectr. Electr. Insul., vol. 25, no. 5, pp. 1798–1806, 2018, doi: https://dx.doi.org/10.1109/TDEI.2018.007191.
E. García-Gonzalo, Z. Fernández-Muñiz, P. J. García Nieto, A. Bernardo Sánchez, and M. Menéndez Fernández, “Hard-rock stability analysis for span design in entry-type excavations with learning classifiers,” Materials (Basel), vol. 9, no. 7, p. 531, 2016. doi: https://dx.doi.org/10.3390/ma9070531.
J. Li, Q. Zhang, K. Wang, J. Wang, T. Zhou, and Y. Zhang, “Optimal dissolved gas ratios selected by genetic algorithm for power transformer fault diagnosis based on support vector machine,” IEEE Trans. Dielectr. Electr. Insul., vol. 23, no. 2, pp. 1198–1206, 2016, doi: https://dx.doi.org/10.1109/TDEI.2015.005277
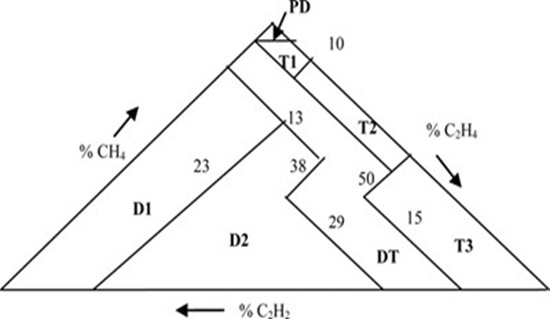